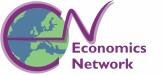
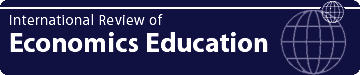
Principles for Teaching Economic Forecasting
David G. Loomis and James E. Cox, Jr
International Review of Economics Education, volume 2, issue 1 (2003), pp. 69-79
DOI: 10.1016/S1477-3880(15)30151-1 (Note that this link takes you to the Elsevier version of this paper)
Up: Home > Lecturer Resources > IREE > Volume 2 Issue 1
Abstract
A forecasting class can be a vital addition to the economics curriculum so that economics graduates will be prepared to meet the demand for forecasting skills required by employers. A previous article (Loomis and Cox (2000)) provides an outline of how forecasting fits into the economics curriculum and what should be taught in an economic forecasting class. Yet recognising the need for such a class and providing the right content is only part of the solution. Proper pedagogy is essential to the success of a forecasting course. This article provides principles on how to teach microeconomic forecasting in a way that will enhance student learning.
JEL Classification: A22
Introduction
Traditionally, economics departments have not offered a stand-alone forecasting class. Most departments offer an econometrics course or a time-series analysis course, but the forecasting content in these courses is extremely limited. The most popular textbooks for econometric courses provide an average of fewer than ten pages on forecasting.
Yet departments have several compelling reasons to add a forecasting class to their curriculum.1 First, forecasting is an important process in most businesses and therefore an important skill to possess as an employee. Second, the demand for professional forecasters is growing, so future graduates will readily find jobs available in this area. Third, a forecasting course complements other quantitative methods courses by providing a more in-depth study of data preparation, information appraisal, empirical judgement and process management. Finally, a forecasting course bridges the gap between theoretical models or abstract statistics and real-world applications by providing a corporate context in which these models and statistics are used. Additional rationale for offering a forecasting course in the economics curriculum can be found in Loomis and Cox (2000).
Since an economic forecasting course is not taught in the majority of economics departments (Hanke and Weigand, 1984), economics faculties may have many questions regarding what should be covered in such a course and how to teach this type of course. We have outlined the content of a one-semester course in a separate article (Loomis and Cox, 2000), so we will simply list a sample syllabus here for clarity (Table 1). We should note that this course excludes macroeconomic forecasting but would provide a more applied and focused complement to a standard econometrics or time-series analysis course. As theory is learned in class, students apply it in a practical situation. We feel it is best to learn the theory in an applied context.
Table 1 Syllabus for an economic forecasting course class
Week | Topic |
---|---|
1–3 | Forecasting taxonomies and process |
4–5 | Evaluation of forecasting techniques |
6–7 | Data collection and preparation |
8–10 | Econometrics/quantitative explanatory forecasting |
11–13 | Time-series forecasting |
14–15 | Qualitative/technological forecasting |
16 | Implementation of forecasting |
In this paper, we present five principles for effectively teaching an economic forecasting class. Many additional teaching techniques could be added to this paper to make teachers better in a general way (see, for example, Becker and Watts, 1995), but the principles we present address specific issues in teaching forecasting.
Each of the five principles we present was derived from over 20 years of classroom experience. The principles shown in this paper are also supported by learning theory and good pedagogy. Although these principles do not represent innovations pedagogically, they are unique in their application to forecasting as a discipline. The recommendations may also be relevant to other empirically based courses such as statistics and applied econometrics. We do not assume anything about the students’ background when entering the forecasting class. Student backgrounds could vary from no coursework to an advanced course in econometrics or statistics.
Because of the nature of the subject matter, economic forecasting should be taught in a very applied, hands-on manner if learning is to be maximised. Rather than having students memorise a list of statistical techniques, students should be taught critical thinking skills in learning to apply different techniques to different data series and distinguish between appropriate uses. Hand calculations and graphical analysis should be incorporated in teaching forecasting techniques and concepts. A forecasting course taught in this manner will encourage the development of skills and proficiencies that all economics majors should have (Hansen, 1986, 2001).
Principles for teaching economic forecasting
Principle 1: Teach forecasting as an applied course with ‘real-world’ relevance
An economic forecasting class should not be taught (as is sometimes done in statistics classes) where students learn methods but fail to see how these methods are actually used in a firm. Forecasting must be put in the context of real business problems. Learning theory supports the idea that real-world relevance is important to students’ motivation and ability to learn. As Saunders (1998, p. 92) states, ‘a perceived usefulness of the material or, even better, an ability to use it, stimulates student interest and intent to learn’. Teaching forecasting with ‘real-world’ relevance helps motivate students and stimulates learning. A forecasting course can easily be taught in a way that emphasises forecasting’s usefulness to the firm, since forecasts are used by almost all areas within the firm (Mentzer and Cox, 1984). Jeff Mello (1997) strongly advocates teaching that focuses on real-world problems. He states, ‘I realized that grades meant little if they weren’t backed up by an understanding of real-world issues that organizations faced and relevant (read: marketable) experience’ (Mello, 1997, p. 179).
Any proper course in forecasting will teach the theoretical foundations. The problem is that many courses stop there and do not demonstrate forecasting’s relevance to the real world. Real-world relevance can be accomplished in several ways. One way is to show examples where forecasting may be used or has been used. Current magazine or newspaper articles that use forecasting can be used as examples in class. Another method is to present case-study applications where businesses have used forecasting in a variety of ways. These can be found by attending presentations of case studies by company forecasters given at the conferences of the International Institute of Forecasters and the Institute of Business Forecasters. Journals such as the Journal of Business Forecasting and Business Economics are another source of actual corporate case studies.
Another way to provide a ‘real-world’ approach is to make a concerted effort to relate each topic covered to how it might practically be used in corporate forecasting. Actual corporate or economic data with accompanying background information can provide added interest as various techniques are discussed and demonstrated. Showing what methods practitioners deem as useful in various applications or giving personal examples from the instructor’s own forecasting or consulting experience can be useful.
In addition, exercises and projects can to be designed to replicate real-world situations. There are a wide variety of jobs that perform forecasting as an integral part of their responsibilities. These include salesperson, product manager, demand analyst, market researcher, comptroller and econometrician. Exercises can be structured to simulate typical job situations where forecasting is used. In addition to simulations, a speaker can be brought into class from a local business or corporation to speak about how they use forecasting. If a live speaker is not available, videotapes of forecasting practitioners can be used.
Another way to provide real-world experience is to use real time-series data. Many websites provide economic time series. For the USA, government statistics can be found at the Bureau of Labor Statistics website (http://www.bls.gov/data/), the White House Briefing Room (http://www.whitehouse.gov/fsbr/esbr.html) and Fedstats (http://www.fedstats.gov/). Economagic (http://www.economagic.com/) provides over 100,000 data files on a free and/or subscription basis. These data can be used on a stand-alone basis or as part of an economic system of equations (for a demonstration of how a real-world experience can be incorporated into a macroeconomic forecasting class, see Donihue (1995)).
Principle 2: Give students ample ‘hands-on’ experience in using forecasting methods
‘Hands-on’ exercises and projects are not the norm in economics pedagogy. According to a recent survey by Benzing and Christ (1997), lecture with the support of blackboard, class discussion and textbook is the predominant teaching method of economics faculties. However, 50% of respondents indicated a movement away from straight lecture style towards increased student participation and interaction. Bartlett and Ferber (1998, p. 117) point out the importance of using ‘hands-on’ experience in teaching:
[A] repertoire of teaching techniques consistent with different learning styles is preferable to using a single one, as is often done in economics. For instance, teaching strategies that give students the opportunity to collect data and to make observations would appeal to the concrete learners; reflective learners would be comfortable interpreting the data; model-building would be the forte of the abstract conceptualizers; and empirical testing would be the preferred mode of those students who like hands-on experimentation.
The Experiential Learning Cycle gives four phases that learning naturally moves through: experience, reflection, abstraction and experimentation (Kolb, 1984). ‘Hands-on’ exercises and projects are essential to allow students to go through each of these phases. A hands-on project will allow a student to be exposed to a forecasting situation and relevant variables (experience), to relate their forecasting knowledge to the forecasting situation (reflection), to formulate the forecasting problem as a forecasting model to be solved (abstraction), and to try different forecasting solutions to see which is most effective (experimentation).
In the forecasting context, it is important to give students in-class and out-of-class ‘hands-on’ assignments to understand the forecasting process and techniques. For example, students could be asked to write a short paper on how a local business generates forecasts. In addition to these shorter exercises, we strongly urge that a more comprehensive, in-depth group project be done to enable students to appreciate more fully the difficulties with doing a larger multi-step forecasting study. This project would expose students to all the teaching strategies mentioned by Bartlett and Ferber (1998) above and lead them through all phases of the Experiential Learning Cycle (Kolb, 1984). This also gives the students a chance to go through the whole forecasting process. This can be done either by using a written case or, better yet, by working on an actual forecasting project with a local business. Working with local businesses shows students the real problems that businesses have in implementing forecasting methods and enhances the real-world relevance of Principle 1. However, it is important that the project not be open-ended but be structured by relating the course material to the students’ practical application throughout the course. Class deadlines for completion of intermediate steps of the project will keep the students on schedule and ensure that the project is manageable. A project outline is shown in Figure 1.
In doing the forecasting project, students can see that there are a lot of judgements to be made in going through the forecasting process. For example, students exercise judgement in selecting the variable(s) to forecast, segmenting the variables, choosing the appropriate time period, deciding which data are relevant and choosing the appropriate parameters in the model. In class, all techniques are covered but projects help the students to decide which techniques are appropriate for a particular project. These judgements are seldom performed in typical textbook exercises.
Figure 1: Forecasting project outline
Section I: Forecasting audit
This section does an audit to find what the current forecasting practices are of the company/organisation. This includes a listing of historical data of the variable to be forecast and graphs of the time-series and explanatory data used in the analysis.
Section II: Analysis and parameterisation
Each technique is run using historical data from the company. Where the technique has parameters, the optimal parameters are found that minimise error. Techniques used in the analysis include: naive methods, moving averages methods, exponential smoothing methods and multiple regression.
Section III: Recommendation of a forecasting technique
A recommendation is given to the company/organisation regarding the best technique to be used for its forecasting. This takes into account the information gathered in the forecasting audit and the analysis that was done in the previous section. The technique that is recommended is demonstrated using the company’s or organisation’s data.
Section IV: Future prospects
Comment is made on factors likely to affect the company’s forecasting in the future. This will give some idea of when the recommended technique might need to be replaced or updated.
Appendix
Anything done to alter the data is explained here: missing values, deflators, adjustments for special events, etc.
Principle 3: Require students (initially) to do hand computations to learn the methods and the interrelationships between methods
With the advent of computer packages, it is possible to have the students read about forecasting and look at computer output but never fully appreciate what a forecasting method is doing. This is sometimes referred to as the ‘black box’ syndrome. It is the authors’ experience that to avoid this syndrome, some hand computation must be done to enable the student to understand first-hand how a forecasting technique operates when the method is initially taught. Hand calculations should be demonstrated in class with additional hand computations performed as homework. Of course, after this is done, computer assistance is a must because it would be too tedious to do all the forecasting work by hand. In order to use forecasting methods effectively, students need a detailed understanding of how the methods work and the underlying assumptions of the technique. Doing hand calculations enables them to see the actual mechanics of the technique. For example, in performing exponential smoothing, students can see the recursive nature of the technique and the role of previous forecasts in the current forecast. Hand calculations add confidence in using the methods, since they know how the techniques work. Students have told the authors that they did not really know how a technique worked until they did the hand calculations.
From learning theory, we know that inductive thinking allows students to formulate ideas from examples and to construct defensible explanations. This is a data-driven, bottoms-up approach (Timpson and Bendel-Simso, 1996, p. 40). There are five steps to inductive study: (1) provide or generate data, (2) separate data into categories and label, (3) analyse interrelationships among categories, (4) develop hypotheses that explain relationships, (5) test hypotheses (Timpson and Bendel-Simso, 1996, p. 42). By requiring students to do hand computations, they can inductively learn the forecasting method used and how it relates and compares to other forecasting methods. Many forecasting methods are related and the interrelationships should be pointed out. For example, single exponential smoothing is a special case of a weighted moving average. Miller (1956) shows that students need this type of organisation and hierarchical categories to structure and support memory because the mind is limited in its capacity to remember discrete bits of information. Confusion will probably result if a student does not get an overview of these interrelationships due to the many different methods that are covered.
Given the numerous forecasting packages that are available, requiring the students to do hand computations also allows more software independence. If students too quickly become dependent on one particular piece of software, they may be hesitant to use a different package. If students understand how to perform the calculations by hand, they will be able to implement some of the simpler forecasting methods on their own using spreadsheets.
Principle 4: Teach students to think critically about their forecast
The need for critical thinking is paramount in the forecasting context. It is our experience that students tend to develop a forecast with easy-to-use software and never take the time to assess the reasonableness of the forecast. Students need to learn to step back and evaluate what the technique is doing and if it is the most appropriate technique to use. Paul (1995) emphasises the importance of critical thinking skills in learning rather than memorising specific facts about a domain of study. Although scholars differ in their exact definition of critical thinking, some notion of self-assessment, judgement and analysis is usually common among the definitions.
The tendency among students is to accept blindly whatever comes out of their model without evaluating it. The thinking of students is that once they have laboured to enter the data, corrected for some outliers and decided on a modelling technique, then their job is done. Whatever comes out of the model must be correct. Students should be taught that critical thinking is crucial in the forecasting process. Students need to critically examine their forecasts on several dimensions including:2
- Is the forecast reasonable based on the historical pattern? (It may be necessary to plot the history and the forecast on the same graph and visually inspect whether the two series go well together.)
- Were the data appropriately preprocessed before a forecasting model was applied?
- Is the technique used appropriate for the forecasting problem? (For example, if there an unusually large price change is projected for the forecasted time period, it is not appropriate to use unadjusted exponential smoothing.)
- Is the technique used appropriate for the data?
- How does the technique rate on other factors besides accuracy (data required, ease of understanding, cost to operate, etc.)?
- How will someone who is unfamiliar with the data view the forecast?
- What supporting information should accompany the forecast to help the user better understand the forecast?
- Are the assumptions used to generate the forecast realistic?
- Should adjustments be made to the forecast based on expert opinion (the forecaster’s or others’), outside information that was not included in the model, and other forecasts generated by alternative techniques?
Principle 5: Use graphs as much as possible
It is common for instructors primarily to use equations without the use of graphs in demonstrating and using forecasting methods. Yet, graphical analysis often provides new insights into the methods and the data. Students are much better at the mathematical details if the technique is first explained in a graphical manner. It helps to have a multimedia classroom where spreadsheet graphs can be projected, but overheads work fine as well. Starting from a simple moving average model, more advanced techniques such as exponential smoothing can be explained by building upon the earlier techniques. For example, a three-month simple moving average can be shown graphically by taking the last three data points and averaging them (by approximating on the graph) and showing where the forecasted point would be. Exponential smoothing can then be motivated by pointing out that the last data point contained much more information about the future than the first data point that was included in the average. Yet a simple moving average gives each data point equal weight in the forecasted value. To account for the additional information contained in more recent data, it would be best to weight the more recent observations more heavily. This is exactly the difference between simple moving average and exponential smoothing. Exponential smoothing can be shown graphically by placing the forecast much closer to the last data point than the simple moving average forecast.
Graphical analysis also helps students who are less proficient in mathematics to visualise these techniques. Intelligent learning shows that if one is teaching mathematics to students who are weak in logical-mathematical intelligence, one can use other intelligences in learning (Gardner, 1983). These intelligences include linguistic, spatial, musical, bodily-kinesthetic, interpersonal and intrapersonal. These could be accomplished by using more visual images, descriptive statements for concepts, or hands-on activities to demonstrate points. For example, graphically showing the effect on the forecast of changing model parameters is more instructive for many students than numerically generating alternative forecasts by changing the parameters in the mathematical equations. This graphical explanation can help spatial learners who are weak in logical-mathematical intelligence.
Students should be taught that graphical analysis is crucial to good forecasting. It is especially important to graph the historical data for the series to be forecasted in order to identify trend, seasonality, cycles or outliers. In regression analysis, it is important to graph both the dependent and independent data to help determine if there is a linear relationship or if there are lead or lag structures. Graphing should be used in illustrating the forecasting characteristics of different methods. By graphing forecasts of alternative methods on the same data, students can compare the methods’ ability to capture various data patterns. This is vital if the students are to learn how to select the appropriate technique for each forecasting situation. Another area where graphing is crucial to good forecasting is in evaluating the effectiveness of data transformations. Graphical analysis vividly demonstrates the effect of transformations and if the transformation has altered the data as intended. Visually seeing the effect of the data transformation helps the student to understand what the transformation has accomplished. Graphing is so important that several textbooks devote a whole chapter or a major part of a chapter to graphical analysis early in their presentation of forecasting (see, for example, Diebold, 1998; Bails and Peppers, 1993).
Conclusion
In this paper, we have examined five teaching principles that will help ensure a successful economic forecasting class. We have advocated a class with real-world relevance and hands-on experience with forecasting methods. Further, we have shown the importance of incorporating hand calculations in teaching forecasting. Critical thinking, assessment and analysis are also important skills to be acquired in learning the forecasting process. Finally, we recommend using graphical teaching methods as much as possible. If these suggestions are followed, we predict that this will enable professors to teach a better forecasting class and students to learn more effectively.
Contact details
David G. Loomis
Associate Professor
Department of Economics
Illinois State University
Campus Box 4200
Normal, IL 61790-4200
USA
Tel: (309) 438-7979
Fax: (309) 438-5228
Email: dloomis@ilstu.edu
James E. Cox, Jr
Professor
Department of Marketing
Illinois State University
Campus Box 5590
Normal, IL 61790-5590
USA
Tel: (309) 438-7286
Fax: (309) 438-5510
Email: jecox@ilstu.edu
References
Bails, D. G. and Peppers, L. C. (1993) Business Fluctuations: Forecasting Techniques and Applications (2nd edn), Englewood Cliffs, NJ: Prentice Hall.
Bartlett, R. L. and Ferber, M. A. (1998) ‘Humanizing content and pedagogy in economics classrooms’, in W. B. Walstad and P. Saunders (eds), Teaching Undergraduate Economics, New York: McGraw-Hill.
Becker, W. E. and Watts, M. (1995) ‘Teaching tools: teaching methods in undergraduate economics’, Economic Inquiry, vol. 33, October, pp. 692–700.
Benzing, C. and Christ, P. (1997) ‘A survey of teaching methods among economics faculty’, Journal of Economic Education, vol. 28, no. 2, pp. 182–8.
Diebold, F. X. (1998) Elements of Forecasting, Cincinnati: Southwestern College Publishing.
Donihue, M. R. (1995) ‘Teaching economic forecasting to undergraduates’, Journal of Economic Education, vol. 26, no. 2, pp. 113–21.
Gardner, H. (1983) Frames of Mind: The Theory of Multiple Intelligences, New York: Basic Books.
Hanke, J. and Weigand, P. (1994) ‘What are business schools doing to educate forecasters?’, Journal of Business Forecasting, vol. 13, no. 3, pp. 10–12.
Hansen, W. L. (1986) ‘What knowledge is most worth knowing – for economics majors?, American Economic Review Papers and Proceedings, vol. 76, no. 2, pp. 149–52.
Hansen, W. L. (2001) ‘Expected proficiencies for undergraduate economics majors’, Journal of Economic Education, vol. 32, no. 3, pp. 231–42.
Kolb, D. A. (1984) Experiential Learning: Experience as the Source of Learning and Development, Englewood Cliffs, NJ: Prentice Hall.
Loomis, D. G. and Cox, J. E., Jr (2000) ‘A course in economic forecasting: rationale and content’, Journal of Economic Education, vol. 31, no. 4, pp. 349–57.
Mello, J. (1997) ‘Teaching in the real world’, in Researchers Hooked on Teaching: Noted Scholars Discuss the Synergies of Teaching and Research, Thousand Oaks, CA: Sage Publishers.
Mentzer, J. T. and Cox, J. E., Jr (1984) ‘Familiarity, application, and performance of sales forecasting techniques’, Journal of Forecasting, vol. 3, no. 1, pp. 27–36.
Miller, G. (1956) ‘The magical number seven, plus or minus two: some limits on our capacities for processing information’, Psychological Review, vol. 63, pp. 81–97.
Paul, R. (1995) Critical Thinking: How to Prepare Students for a Rapidly Changing World, Santa Rosa, CA: Foundation for Critical Thinking.
Saunders, P. (1998) ‘Learning theory and instructional objectives’, in W. B. Walstad and P. Saunders (eds), Teaching Undergraduate Economics, New York: McGraw-Hill.
Timpson, W. M. and Bendel-Simso, P. (1996) Concepts and Choices for Teaching: Meeting the Challenges in Higher Education, Madison, WI: Magna Publications.
Notes
[1] We recognise that there are time constraints and budgetary constraints that require trade-offs when offering new courses.
[2] As students gain more knowledge throughout the semester, their critiques of their forecasts will become better. However, several of these questions can be asked and answered with very little knowledge of the underlying techniques. We believe it is important for the students to think critically about their forecasts very early in the course.