Dr Tim Burnett
Aston University
t.burnett at aston.ac.uk
Part of the Virtual Symposium on Online Teaching Published June 2020
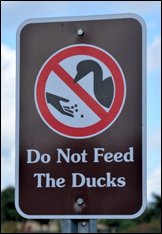
Do Not Feed The Ducks! Using self-guided data exploration in Excel to facilitate discovery, promote engagement, build skills, and break the cycle of student dependence
Before you begin, ask yourself two questions:
Think about the last time you learned how to do something (perhaps in Excel, or Stata); how did you learn it?
Think about a skill you’re good at (e.g. playing a musical instrument, or writing) how did you get good at it?
1. Introduction
1.1 A reflective pause on why we should think about the way we teach
It is important to take a moment to consider what we want to achieve through education and how our teaching design impacts on learning outcomes—both in terms of skills, and the behaviour of graduates.
The typical design of an economics module consists in introducing a topic in lectures, accompanied by a description of the prevalent theories economists have put forward for understanding the characteristics of the topic. This situation is rarely reflective of life outside of academia (such as in employment), where we encounter or ‘discover’ problems (topics, issues) first, and then must identify the best way to address or understand them.
1.2 The present role of data in economics education[1]
There is clearly an almost infinite variety of ways in which economics is, and can be, taught—including the way that teaching incorporates data. However, there are two very common patterns:
Quantitative education and theory are taught separately: Teaching quants as separate from economics is widespread, with linkages between the two limited to examples or datasets which are provided to quants students. Theory, on the other hand, is taught using abstract examples (e.g. “let’s say the demand curve for apples is…”)—this introduces an immediate barrier between observable reality and theory being taught.
Quantitative data is provided to validate a theory that has already been explained to students: It is not uncommon to see students provided with a dataset which will be discussed in a small-group tutorial/seminar/workshop. This approach is usually employed to demonstrate that a theory holds in the ‘real world’.[2]
This case study proposes a deviation from these strategies.
1.3 A different way to use data in economics education
The (seemingly uncontroversial) proposal detailed in this case study is that students should be given relevant data before taught lectures and encouraged to explore the data for themselves. This idea, and the benefits which stem from it, rest on two key features:
Students are provided with time and space to explore the data themselves with minimal intervention—it should be the students doing the work
This process of investigation is repeated such that students become accustomed to the process of examining data to discover patterns, relationships, etc.
Although this seems like a relatively small change to make to our teaching, I argue below that this ‘data-first’ approach has substantial benefits across a range out outcomes. This is followed by a set of practical guidelines for implementation, and, finally, several examples drawn from economics teaching.
The following section outlines the theory which underpins the proposed approach, before guidelines for implementation are introduced, accompanied by a number of examples.
2. The theory behind the approach
2.1 Why is important that students do it for themselves?
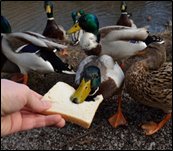
Conventional teaching as described in Section 1 can create expectations/dependence in students that all the information they need will be conveyed to them by their lecturers—in effect, training students to be good listeners, but not active and engaged learners. This process of knowledge acquisition as turning up, listening, and taking in information, is markedly different from the way researchers (or others) acquire knowledge through active engagement and ‘seeking’ of answers.[3]
On the contrary, when we ask students to dig out and ‘discover’ things for themselves (as I propose), they become much more active participants in learning, and must engage with specific skills of creativity, judgement, and decision making (amongst others). These more ‘constructivist’ or ‘experiential’ learning approaches (such as the present example of providing students with data, but minimal scaffolding, from ‘day-one’) promote a very different set of learning skills and outcomes relative to the case where students are ‘told’ things to remember.[4]
This added engagement with material, and the exploration in which students have engaged, means that lectures where theory is explained can be much more participatory, active environments. Students will have observed the data and even explored it, they may have ‘discovered’ relationships or patterns. It is in fact the ‘discovery’ element that becomes the central foundation of a taught class—helping students apply theory to a topic which they have explored through data first.
Moreover, as outlined below, when students are asked to repeat this process this results in a broad range of benefits. Section 4 provides some specific examples where this process (data first, theory second) either has been, or can be, implemented.
2.2 Why is it important that it is repeated?
“…coming to know, and especially being able to use knowledge and skills generally, requires reinforcement, application, repetition, and often practice in a variety of settings and contexts in order for it to become fully understood, integrated, and accessible in future situations.”
– Ruben (1999), p.499
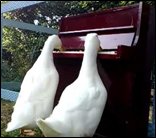
It is important that the data-first process is not a one-off and that students can practice, through repetition, the process of exploration and skill acquisition.
That practice and repetition results in competence is not revolutionary. Indeed, in order to prepare for exams, we frequently provide students with practice problem sets and exam questions, so they can become competent at addressing the type of questions they will face. We are, however, less willing to countenance or act on the idea that other desirable skills (such as communication, resilience, or initiative) can also be trained through repetition; the example of the once per year presentation providing ‘employability skills’ springs immediately to mind.
The repetition of self-directed data exploration has a number of beneficial outcomes for students, both academically and in terms of employability:
As detailed above, as students adapt to model of helping themselves, and are weaned off a model of education where all content and skills are taught, this promotes a self-reflective ability to identify skills and knowledge shortcomings, and a willingness and ability to independently act to remedy these. Developing students’ confidence in their own ability to deal with problems has clear implications as students are confronted with increasing complexity as their courses progress.
A willingness and ability to adapt and autonomously respond to challenges makes students more employable. While the term ‘employability skills’ is not uncontested, the employability responsibilities of HE institutions were recently put forward by Jenkins and Lane (2019) as:
“Higher education should prepare students to get a good graduate-level first job and help them to develop skills to enable them to succeed at work and in their wider life”
Which lends itself to a relatively uncontroversial definition of employability skills as ‘skills which will help a graduate gain a job, and then to succeed at that job’.
A key issue with the idea of ‘work ready’ graduates equipped with sufficient skills to ‘hit the ground running’ is that this conflicts with the notion that we, as economics educators, are keen to emphasise to students that economics degrees can lead to a range of different career options. The broader a range of graduate destinations, the harder it is to teach graduates the ‘right’ set of skills (in economics parlance, it becomes a matching problem). Different career destinations will each have their own set of desired skills and competencies. However, encouraging students to seek answers and ‘discover’ economics for themselves builds creativity, initiative, and judgement. Equally important is that, by requiring students to reflect on their skills and acquire for themselves appropriate tools and knowledge, students become more proficient at adapting—gaining resilience and flexibility.
2.3 Why use Excel?
There are a number of compelling arguments why Excel should be favoured over more involved statistics packages like R or Stata:
2.3.1 Basic data skills and the importance of descriptive statistics
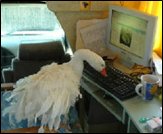
An extraordinarily common occurrence in undergraduate (or all?) empirically-based work is the tendency of students to undervalue the importance of descriptive statistics and data visualisation (McIlroy, 2003). This extends both to a shortage of space and time devoted to their exposition within work, and also to a general lack of critical engagement with the implications of various headline statistics or distributions.
Requiring students to engage with Excel, and its different set of headline features relative to statistical analysis packages, promotes engagement with those areas where Excel dominates—visualising data, sorting and filtering, and production of basic granular descriptive statistics.
2.3.2 Tendency of students to over-complicate when using Stata
One common trend that many quantitative educators will have observed, is the tendency of students to skip about 17 steps when analysing data using Stata. Students, emboldened by the knowledge of Stata syntax and the capabilities of the software, demonstrate a proclivity to immediately and uncritically embark on linear regression analysis (or even more complex approaches) without having first ‘looked’ at the data.
This is not just a procedural annoyance. Understanding distributions and the nature of data is key to the development of appropriate model specifications and selection of technique. Using Excel forces students to engage with these aspects of data.
Economics students, inculcated into an echo chamber of complex statistical software and conversation, easily lose track of the reality that non-economists have no idea what linear regression analysis is. Using Excel keeps students engaged with a more ‘accessible’ level of data analysis.
2.3.3 Accessibility for all cohorts
It is known that elective economics modules are studied by students from across the university, particularly in related fields such as sociology, politics, or international studies. Many of these students will not be studying intermediate or advanced statistics throughout their degree, thus using Stata or SPSS can unconsciously exclude these students.
2.3.4 Employability
Last, but not least, recent research (Jenkins, 2019) has suggested that, despite a general level of satisfaction with economics graduates’ quantitative skills, employers still have concerns over the ability of graduates to use Excel. Excel is ubiquitous and employed at all levels of most major organisations. The tendency of economics degrees to focus on quantitative education via Stata, R, or SPSS (mentioned above in Section 2.3) occurs at the cost of normalising the use of Excel.
3. Implementing a ‘Data-first’ approach
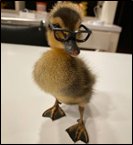
The implementation of a “data-first-theory-then” approach to teaching does not necessarily require extra teaching time (though this can be accommodated, depending on implementation). I have divided the implementation in three steps:
3.1 Finding or Simulating data
The main ‘cost’ involved in including an exploratory data stage in economics education is the time cost of collecting or simulating data. The data that is given to students needs to be directly relatable to the subject of the class, so in some cases there may be relevant data which is readily available (such as in the example 3 in Section 4), but in others the easiest strategy may be to simulate some plausible data (example 2)—either from scratch or from a ‘nub’ of a dataset.
3.1.1 Uhm… Simulated data?[5]
Because the object of the ‘data-first’ approach is to encourage students to engage with data autonomously, there is a strong case that it matters not whether the data is real or not. In both cases, there is a process of investigation and ‘discovery’. Just keep in mind that, whilst simulated datasets can be really powerful tools, we always need to be vigilant to the possibility that simulated data might leave students with misleading ideas about reality. With this in mind, it is always important to ensure that simulated data is plausible and rooted in reality, to be transparent about the source of data and how datasets are constructed, and to fully debrief students about the data they have used.
3.2 Challenging students
You should issue the dataset to students in advance of the lecture, preferably with plenty of time for students to look into it. If scheduling permits, you may wish to issue the data in a classroom environment, such that students can work together.[6] It is vital that this ordering is maintained —providing data-based tasks after lectures eliminates all the potential learning associated with discovery and exploration.
In conjunction with the data, you have to provide students with a set of guiding questions. These questions should not have a defined or definite answer, but should be worded as ‘prods’ to encourage students to look at particular aspects of the dataset. Examples might include:
- Do you notice anything about the relationship between X and Y? Why do you think this might be?
- What does the variable X look like? What do you think are the implications of this?
Note: Both the questions above are asking students to reflect, and to use judgement. You will later have time to fill in theoretical details, or correct misconceptions, but, for now, giving students breathing space to think and explore is vital.
A key point here, also, is to ensure that you do not provide too much context to students; the more context is provided, the more students will tend to fall back on knowledge or heuristic ideas which might impact how they go about the task. Don’t use phrases like “theory tells us…” or “many people think…”—these create unwanted focal points, when we are truly interested in de-anchoring students and allowing them to express creativity.
3.3 Scaffolding and crib sheets
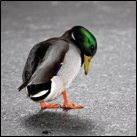
Resist the urge to tell students how to do everything!
By expecting students to find their own answers you are not failing them. On the contrary, describing how students should do everything actually creates a ‘dependency’. Like ducks who have been refused a bit of sandwich, students will complain, give ‘sad eyes’, perhaps even mark you down in module evaluation[7]—this is a normal part of breaking a cycle of dependence.
That said, insofar as this piece is advocating “leaving students to their own devices” (only at the beginning, plenty of support afterwards), pragmatic necessity dictates that there is nothing wrong with giving them a head start. You may wish to start out with a general ‘Getting started with Excel’ worksheet which introduces them to some of the capabilities of Excel or signpost them towards a useful online video (or one created by yourself).
In order to align activities with the desired outcome of creating self-regulating learners, any help you do provide should be focussed around building capability, focussing on developing students’ skills of self-sufficiency, rather than providing step-by-step guidance on how to carry out specific tasks.
3.4 How to deal with ‘hard’ skills?
A common complaint which is levelled at educators who advocate a hands-off approach to experiential learning (such as Problem-based Learning) is that students may acquire critical skills and the ability to apply subjectivity and judgement, but there is no guarantee that they acquire necessary ‘hard’ skills (which in this case would be the ability to effectively use the wider capabilities of Excel).
Obviously, students will need to establish some skills, but actively working with data gives students the opportunity to learn as they go along — reflecting, identifying, and remedying knowledge and skills shortcomings as required. This is where judiciously-worded guide questions and signposting can help. For example, you might want to highlight to students effective and reliable ways of finding Excel help online or how to use Excel’s inbuilt help function (helping students to help themselves). You may, at the start want to demonstrate to students how to enter a formula in a cell, but you don’t need to tell them every formula they need—the same applies to producing graphs and visualisations. Students don’t need to be told everything, and with the right support they should be able to start working things out for themselves, as we do when carrying out research.
3.5 Linking data to theory
The last step, once students have been provided time and space with the data, is to use the data as the starting point to theory-based lectures. Students should be permitted a meaningful voice in these classes, and you should begin a class by asking students what they noticed in the data (as informed by any guide questions you issued). This stage is really important as it legitimises the efforts students have made in trying to understand the data.
3.5.1 Correcting students
It is quite important that, as an educator, you do not simply dismiss the findings of students, even if their answer is not correct or what you expected. Unlike in conventional ‘homework’, you are not setting a question which has a defined answer—you are asking students what they think. Ask students how they got to the answer, ask them the implications of their answer. Perhaps introduce some theory and return to their answer(s). If their answers don’t match the theory, ask them why they think this might be and remember that theory is just that; a theory. In most cases it is a mathematical formula which has been put forward to explain economic phenomena—these are perfect circumstances to help students understand this, and to use data to be critical of theory which is presented.[8]
3.6 Adaptation to remote learning
As a method of priming students for further, specific, learning, data discovery tasks make an ideal addition to a remote or blended learning approach. They can be carried out remotely in students’ own time. Because data-first approaches can improve engagement, this approach can do a lot of the ‘heavy lifting’ for you in terms of stimulating student interest in a theoretical topic.
One challenge which may be faced is the ‘debrief’ element which would have taken place at the start of the theory lecture. Because it is productive to have this as a participatory environment, this may be difficult to replicate as distance learning. In this case it may be useful to consider some additional form of engagement, such as a discussion board or forum.
4. Case studies
Below are three case studies where a data-first approach either has been employed (examples 1 and 2) or can easily be employed. All three of these examples are accompanied by datasets.
4.1 Example 1: Using exercises and examples from CORE’s ‘Doing Economics’ resource
CORE’s ‘Doing Economics’ project (which introduces a number of datasets and asks students to carry out various data related tasks) is both an excellent source of datasets, and also features task sheets which ask students to carry out a range of data-related tasks (using Excel or R).
The Doing Economics task sheets can form an ideal basis for crib sheets to encourage students to think about the data, however be wary of the amount of information which is provided to students—this detail can always be filled in later. For example, the questions for Project 3 (which concerns the introduction of a sugar tax) emphasises that this is an area which has been studied (with references) and outlines the use of Difference-in-Difference approaches (DiD) (by name); DiD is an appealingly simple concept for students to get their heads around, so can we gently encourage students to implement DiD without mentioning the approach by name?
On the other hand, the first Doing Economics project was adapted for an interdisciplinary quants-based module at University of Warwick[9] with minimal changes and, once students had autonomously studied the exercise, formed the basis for a classroom discussion on what data can tell us about climate change. This project was particularly helpful as it features some links to tutorials to encourage a learning-by-doing approach—though you may wish to encourage students to search for these themselves.
4.2 Example 2: Using simulated data: Loans and interest rates
This example (Excel spreadsheet provided) was provided to students in advance a class in the same quants module, as mentioned above. This data was simulated to represent the unsecured loan sheet of a high street bank. Students were asked a number of guide questions to help them think about several issues which would be discussed in the following class such as depreciation of assets, wages and loan eligibility, and gender bias and access to credit (note: in keeping with literature, there wasn’t strong evidence in the dataset) (the crib sheet for this dataset is included with this case study).
In the subsequent discussion class a discussion was held about the fact the data was simulated, why a simulated dataset had been used, and how the results of simulated data could be verified against real-life through academic literature.[10]
4.3 Example 3: Using data-first to introduce Okun’s Law
Okun’s Law is an observed empirical relationship between national output and unemployment and can be expressed as:[11]
Δut = α + β(GDP growtht)
Clearly, it is not particularly complex, and students intuitively understand how this operates. But how should we teach it?
In my experience this relationship has been taught in lectures—students are taught about the relationship, then, in subsequent tutorials, students were provided data and asked to verify that the relationship existed and to estimate its parameters. Clearly, however, Okun’s Law is not complex, and students can readily acquire the necessary skills to at least start understanding that there is a relationship before this is verified in theory. Okun’s himself found this relationship looking at the data!
This is a particularly empowering example. Okun’s Law, like many economic ideas is not intrinsically complicated. Students who establish a relationship for themselves will see that they have actually succeeded in identifying a relationship which is relied upon by central banks etc.
5. Student Feedback
The above applications were ‘baked’ into the design of the modules, and many of the benefits associated with the method are fairly nebulous, meaning that definitively measuring their positive impact is difficult. That said, there are several observations which can be made
5.1 Student self-belief
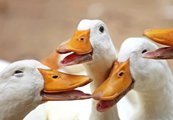
In almost all course where data-first approaches have been employed, students will initially begin the course expressing exasperation, frustration, and a belief that they are not receiving the necessary support. As stated in section 2.1, this is to be expected when removing concrete scaffolding from students’ learning. In all cases, listening to students’ ideas in class, encouraging them, feeding back on what they found, and, thus, providing them with a voice, begins to increase both their confidence in what they are doing, and also the idea that you, as an educator, care about what they have ‘discovered’ or created.
Often these approaches are accompanied by a periodic, partial, ‘drawing back of the curtain’. mentioning to students that what they are doing has real value, that it’s ‘by design’, reminding them what you’re trying to achieve, and (where applicable) the progress that students’ have made. This latter point regarding progress is particularly powerful as it highlights to students that they have progressed and this was achieved not through your teaching, but by their own self-discovery.
5.2 Trust
An interesting (but difficult to measure) externality stemming from this approach is the tendency of students to eventually buy into what you are trying to achieve. In numerous classes where student autonomy is encouraged, student feedback has demonstrated a ‘trust’ in what you, as an educator, are attempting; things which don’t quite ‘come off’ in class (such as classroom experiments which fail to achieve their aims) become a matter of misfortune, rather than a failing on your part. I perceive this as a by-product of the inclusion of students as active partners in learning, and as a form of reciprocity for the trust you place in them.
5.3 Learning outcomes
Having taught quantitative subjects for many years before adopting these approaches, I have not identified any negative impact on subject-specific learning, relative to more conventional approaches.
6. Summary
This case study introduced the idea that students should be provided with economic data and allowed to explore economics relationships before these are formalised in taught theory lectures. Literature on experiential learning suggests that this approach can be identified with improved self-regulation, creativity, judgement, and confidence when dealing with new problems.
Sources and further reading
Barrows, Howard S. (1988) The Tutorial process (Revised Edition). Southern Illinois University School of Medicine, Springfield, Illinois, US
Jenkins, C., and Lane, S. (2019) ‘Employability Skills in UK Economics Degrees: Report for the Economics Network’ [online]. The Economics Network. (Accessed: 17th March 2020)
Ruben, Brent D. (1999) "Simulations, Games, and Experience-Based Learning: The Quest for a New Paradigm for Teaching and Learning." Simulation & Gaming, 30(4), p. 498-505 https://doi.org/10.1177/104687819903000409
Savin-Baden, Maggi (2004) Foundations of Problem-Based Learning. Open University Press, UK
Notes
[1] This is, admittedly, a generalisation and there are numerous ways in which data is incorporate into the curriculum.
[2] I also think the use of the term ‘real-world’ should be avoided as it immediately places material not labelled as ‘real-world’ as belonging in the non-real or made-up world.
[3] This is a generalisation and there are many examples of amazing and engaging teaching in Economics. Whoever reads this is likely already doing an amazing job. In which case, as is usual, I will be preaching to the converted.
[4] Because of the ordering of content, and the hands-on nature of this proposal, there are some parallels here with (amongst others) Problem-based Learning, and Discovery Learning.
[5] While the practical aspect of constructing simulated stands on the periphery of the scope of this case study, I’m always happy to talk about this—just drop me an email at t.burnett@aston.ac.uk
[6] If you do this, then you can add ‘communication’ to the list of skills students will practice and normalise.
[7] Note that ducks are rarely, if ever, involved in university module evaluation exercises—so we can only infer that they might give poor feedback scores.
[8] Barrows (1988) provides an excellent overview to these types of classroom interactions.
[9] IP110 Quantitative Methods for Undergraduate Research deals with the acquisition of quantitative skills but emphasises the interpretation of data through the use of discussion-based workshops.
[10] You can think of this as ‘drawing back the curtain’. In general, I find it perfectly fine to be clear with students about what they’re doing—this approach treats students as adults.
[11] CORE Economics, Section 13.
↑ Top